The Multi-agent system and deep reinforcement learning research team from the School of Software of Tianjin University, based on collaborative research with the University of Tokyo and the National Institute of Advanced Industrial Science and Technology (AIST), for the first time used deep reinforcement learning methods to detect defects in the models of Cyber-Physical Systems. The paper on the collaborative research was adopted by the International Symposium on Formal Methods (FM2018).

With the advent of AlphaGo, the first artificial intelligence program able to beat the world champion of Go, a wave of deep reinforcement learning has set off worldwide, and many scientific research institutions and college research teams at home and abroad have invested in it. Deep reinforcement learning methods have achieved great outcomes in games, intelligent robot control and other fields, such as the development of StarCraft, the robot Atlas and so on.
At the same time, the Cyber-Physical System, a multidimensional and complex system that carries next-generation intelligent technologies such as the Internet of Things, smart homes, robots, and smart navigation, has gradually entered people's lives. However, the security of the system has always been questionable. How to detect defects in the system more efficiently and accurately so as to ensure its stability and security has become the focus of researchers.
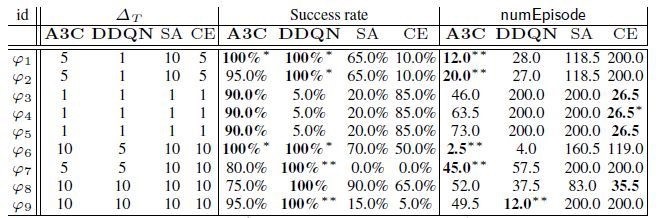
After several years of in-depth research, Tianjin University researchers applied the deep reinforcement learning method to the fault detection of Cyber-Physical Systems for the first time, improving the success rate of system fault detection, and the detection efficiency has also been significantly improved.
The traditional defect detection method is based on robustness (system stability) and adopts various kinds of stochastic global optimization algorithms to minimize robustness. Traditional methods must complete the entire simulation to give feedbacks. Therefore, a large number of repeated simulation operations that take a lot of time are required in the defect detection process, and the test results cannot be guaranteed.
The defect detection method of CPS models based on reinforcement learning adopts A3C and DDQN, the most advanced reinforcement learning technologies in the world, to solve the problem of falsification of some properties for CPS models. The technology can observe environmental feedback, self-optimize, timely adjust the input, and detect system defects with fewer simulations.
By: Qiu Ya
Editors: Qin Mian and Christopher Peter Clarke